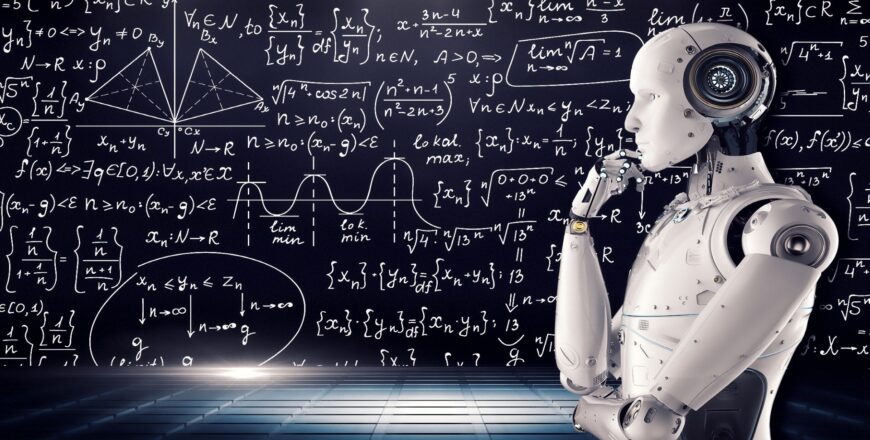
• Introduction to AI: Defining AI, machine learning, and deep learning.
• Types of AI: Supervised, unsupervised, and reinforcement learning.
• History and Evolution of AI: Key milestones and breakthroughs.
• AI Applications in the Real World: Examples of AI in various domains (e.g., healthcare, finance, self-driving cars).
• Supervised Learning:
o Regression, classification algorithms (linear regression, logistic regression, decision trees, support vector machines).
o Model evaluation and selection (metrics, cross-validation).
• Unsupervised Learning:
o Clustering algorithms (k-means, hierarchical clustering).
o Dimensionality reduction techniques (PCA).
• Hands-on Exercise:
o Implementing simple machine learning models using Python and a library like scikit-learn.
• Introduction to Deep Learning:
o Neural networks, deep neural networks, and their architectures.
o Convolutional Neural Networks (CNNs) for image recognition.
o Recurrent Neural Networks (RNNs) for natural language processing.
saturncloud.io
• Deep Learning Frameworks:
o Introduction to TensorFlow and PyTorch.
o Hands-on exercise: Building and training a simple neural network.
• Natural Language Processing (NLP):
o Text classification, sentiment analysis, and machine translation.
o Introduction to NLP libraries (e.g., NLTK, spaCy).
• Computer Vision:
o Image classification, object detection, and image segmentation.
o Applications of computer vision in various domains.
• Reinforcement Learning:
o Introduction to reinforcement learning concepts.
o Applications of reinforcement learning (e.g., game playing, robotics).
• Ethical Considerations in AI:
o Bias in AI algorithms, fairness, and accountability.
o Privacy and security concerns.
o The societal impact of AI.
• The Future of AI:
o Emerging trends and technologies in AI.
o The role of AI in various industries.
o Career paths in AI and machine learning.