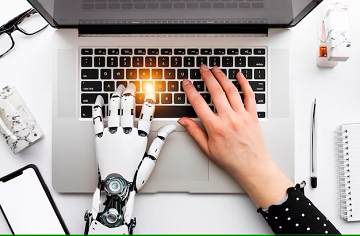
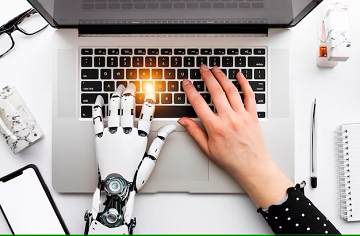
This program equips supply chain professionals with the knowledge and foresight necessary to leverage machine learning (ML) for optimizing operations, building resilience, and preparing for the future of logistics. By incorporating future studies methodologies, participants will gain a strategic understanding of how ML can address current challenges and anticipate potential disruptions within the supply chain landscape. Through a blend of interactive lectures, case studies, hands-on workshops, and group discussions, this program will empower you to develop and implement a future-proof ML strategy for your supply chain.
• Analyse the impact of future trends on the development and application of machine learning in supply chain management.
• Identify key challenges and opportunities within current supply chain operations, considering how ML can address issues like demand forecasting, inventory management, and risk mitigation.
• Develop and implement a strategic vision for integrating machine learning into their supply chain strategy to improve efficiency, reduce costs, and enhance customer service.
• Explore various machine learning algorithms and techniques applicable to different supply chain functions, such as demand forecasting, anomaly detection, and route optimization.
• Gain practical experience using industry-standard tools and platforms to build and deploy simple machine learning models for supply chain tasks (basic coding skills recommended but not mandatory).
• Communicate effectively about the potential and limitations of machine learning to stakeholders within the organization.
• Develop a future-oriented perspective on ML in supply chains and identify potential disruptions and opportunities related to emerging technologies.
• Create a personalized action plan outlining steps to implement ML solutions within their specific supply chain function.
• Supply chain managers, directors, and planners seeking to optimize logistics processes with machine learning.
• Procurement specialists interested in using ML for smarter sourcing and supplier management.
• Inventory management professionals looking to leverage ML for demand forecasting and optimization.
• Logistics and transportation professionals interested in exploring ML applications for route optimization and fleet management.
• Anyone in a supply chain role wanting to understand the future potential of ML and its impact on their work.
• Pre-assessment
• Live group instruction
• Use of real-world examples, case studies and exercises
• Interactive participation and discussion
• Power point presentation, LCD and flip chart
• Group activities and tests
• Each participant receives a binder containing a copy of the presentation
• slides and handouts
• Post-assessment
Day 1: The Future of Supply Chains & Machine Learning
• Welcome and program overview.
• The Evolving Landscape of Supply Chains: Exploring how technological advancements, globalization, and changing customer expectations are impacting the future of supply chain management.
• Introduction to Machine Learning: Learning the core concepts of machine learning, different algorithms, and how they can be applied to solve problems in supply chains.
• Future Studies for Supply Chain Professionals: Discovering how to incorporate future studies methodologies like scenario planning and horizon scanning to anticipate potential disruptions and opportunities related to ML in supply chains.
• Case Study: analysing a real-world example of a company that successfully leveraged machine learning to address a specific challenge within their supply chain.
Day 2: Machine Learning Applications in Supply Chain Functions
• Demand Forecasting: Exploring how machine learning algorithms can be used to analyse historical data, identify trends, and predict future demand for products, leading to improved inventory management and production planning.
• Inventory Optimization: Learning how ML can optimize inventory levels to minimize costs associated with holding excess stock or stockouts, considering factors like lead times and demand fluctuations.
• Risk Management and Anomaly Detection: Discussing how machine learning can be used to identify potential disruptions and anomalies in the supply chain, such as supplier delays or transportation issues, allowing for proactive mitigation strategies.
• Hands-on Workshop: Demand Forecasting with Machine Learning: Participants will gain practical experience using a user-friendly platform or introductory coding environment to build a simple machine learning model for demand forecasting on a pre-prepared supply chain dataset.
Day 3: Machine Learning for Procurement & Logistics
• Supplier Management & Sourcing Optimization: Exploring how machine learning can be used to analyse supplier performance data, identify the most reliable partners, and optimize sourcing strategies.
• Route Optimization & Transportation Management: Learning how ML algorithms can be applied to optimize delivery routes, considering factors like traffic patterns, fuel efficiency, and real-time data to improve efficiency and reduce transportation costs.
• Warehouse Management & Automation: Discussing the role of machine learning in warehouse automation, including tasks like demand-driven product placement and predictive maintenance for equipment.
• Guest Speaker: A supply chain leader who successfully implemented ML solutions in their organization can be invited to share their insights and answer participant questions.
Day 4: The Future of ML in Supply Chains
• Emerging Technologies & Disruptions: Exploring potential future trends like autonomous vehicles, blockchain technology, and the Internet of Things (IoT), and how these might further impact the application of ML in supply chains.
• The Human-Machine Collaboration: Discussing the future of work in supply chain management with the integration of ML, focusing on the evolving role of human expertise and decision-making alongside automation.
• Ethical Considerations & Data Governance: Addressing the ethical challenges associated with using machine learning in supply chains, such as bias in algorithms and data privacy concerns.
• Case Study: analysing a real-world example of how a company is preparing for the future of ML in their supply chain by adopting responsible AI practices and building a culture of data literacy.
Day 5: Building Your ML-Powered Supply Chain Strategy
• Developing an ML Implementation Strategy: Creating a roadmap for integrating machine learning into your supply chain operations, considering factors like data infrastructure, talent development, and stakeholder buy-in.
• Identifying ML Use Cases: Participants work in facilitated groups to identify specific opportunities within their own supply chain functions where ML could be applied for optimization and improvement.
• Evaluating & Selecting ML Solutions: Learning strategies for evaluating and selecting appropriate machine learning solutions based on specific needs, functionalities, and costs.
• Building an Action Plan: Participants create personalized action plans outlining steps to explore and implement machine learning solutions within their specific supply chain role or department. This may include:
o Identifying key performance indicators (KPIs) to measure the success of ML initiatives.
o Researching available tools and resources for building or deploying ML models.
o Advocating for responsible and ethical practices in ML implementation within your organization.
• Course Wrap-Up & Ongoing Learning: Reviewing key takeaways from the program, addressing any remaining questions, and discussing ongoing learning resources for staying informed about advancements in machine learning and its application in supply chains.
• Networking & Knowledge Sharing: Participants engage in a facilitated discussion to share their ML action plans, best practices, and potential challenges, fostering collaboration among supply chain professionals.
This site uses cookies. Find out more about cookies and how you can refuse them.